Tables of Content
1. Introduction
Additionally requiring physical labour, audio labelling frequently uses software for annotation. Audio annotation is different from audio transcription, which turns spoken words into written form.
For machine learning techniques and applications, audio annotation is the process of labelling or tagging audio data with descriptive information. It entails adding additional information, such as transcriptions, timestamps, speaker identification, sentiment analysis, or other pertinent metadata, to the audio recordings.
Speech recognition, natural language processing, audio categorization, voice assistants, and other fields all rely heavily on audio annotation. Researchers and developers may teach machine learning models to precisely read and comprehend spoken language or audio material by adding relevant annotations to audio data.
One of the most common types of audio annotation is transcription It entails converting verbatim text from audio files of spoken speech. Keyword searches, content indexing, and spoken content analysis are all made possible via transcriptions. Furthermore, timestamping is utilized to pinpoint the exact moments when particular phrases or events take place in the audio, enabling accurate analysis and referencing.
Another crucial component of audio annotation is speaker recognition. It entails giving each speaker in a discussion or audio recording its own labels. It is feasible to distinguish between individual speakers, keep track of their contributions, and conduct speaker-specific analysis by associating speakers with distinctive identifiers, such as speaker numbers or names.
Sentiment analysis is a useful annotation method for deciphering audio’s subjective or emotional content. Sentiment analysis is a technique that aids in the analysis of customer feedback, voice-based surveys, and audio recordings on social media by recognizing and categorizing the emotions represented in the audio, such as happiness, sorrow, anger, or neutrality.
Additionally, labelling certain audio occurrences or classes may be included in audio annotation. For instance, audio data may be tagged with tags indicating the presence of particular sounds, such as dogs barking, automobile horns, or musical instruments, in audio classification tasks. This makes it possible to create applications for audio-based surveillance, environment monitoring, and sound recognition systems.
In conclusion, audio annotation is an important step that improves the usability and comprehension of audio data. It enables audio material to be effectively understood and analyzed by machine learning models, supporting a variety of applications from sentiment analysis to audio categorization and voice recognition. Audio data may be made more available, searchable, and useful for a variety of companies and research sectors by adding metadata and annotations.
Any audio production should have annotations. It is a strong tool with a wide range of further applications. It can enhance the precision of voice recognition systems, offer more accurate translations, and aid in producing more realistic synthetic speech, among many other advantages.
However, it also has certain drawbacks, such as the requirement for excellent audio recordings and the possibility of incorrect annotation.
An essential step in data analysis is labelling, also known as annotation, which entails giving each occurrence of data a meaningful tag or category. Labelling is essential for activities like voice recognition, speaker identification, sentiment analysis, transcription, and sound event detection when dealing with audio data. It is feasible to derive useful insights, enhance the functionality of machine learning models, and allow a variety of applications by precisely labelling audio data.
Several phases are involved in labelling audio data, including data collection, annotation rules, annotation techniques, and quality control checks.
An essential step in data analysis is labelling, also known as annotation, which entails giving each occurrence of data a meaningful tag or category. Labelling is essential for activities like voice recognition, speaker identification, sentiment analysis, transcription, and sound event detection when dealing with audio data. It is feasible to derive useful insights, enhance the functionality of machine learning models, and allow a variety of applications by precisely labelling audio data.
Several phases are involved in labelling audio data, including data collection, annotation rules, annotation techniques, and quality control checks.
Depending on the difficulty and size of the work, several annotation techniques may be used. Manual annotation entails having human annotations listen to the audio recordings and give labels in accordance with predetermined standards.
Although manual annotation guarantees that the audio data is understood and interpreted at a human level, it can be time-consuming and expensive, especially for big datasets. The labelling process can also be assisted by or partially automated using automated techniques like automatic speech recognition (ASR) or speaker diarization algorithms, which lessens the workload on human annotators and increases efficiency.
In order to guarantee the correctness and dependability of the labelled data, quality control techniques are essential. To find and fix any labelling mistakes or discrepancies, these methods entail validation and verification procedures. When several annotators independently identify the same collection of audio data, for instance, an inter-annotator agreement may be determined, and disagreements are settled by adjudication or debate. To maintain high-quality labelling and solve any possible difficulties that may occur throughout the process, regular monitoring, feedback, and training for annotators are vital.
Labelling in the context of voice recognition is turning uttered words into written text. This project makes it possible to create automatic speech recognition systems, which let computers search, index, and comprehend audio files. The transcribing process calls for more than just precision.
The process of transcribing involves not only precisely recording the spoken words but also overcoming a number of difficulties, including managing disfluencies (such as pauses or repeats) and recognising unique symbols or non-speech sounds.
Identifying the speakers in an audio file is another crucial component of labelling. To identify between multiple speakers within a recording, it entails annotating audio data with distinctive identities or names. In applications like forensic analysis, speech biometrics, or speaker diarization systems, where comprehending or monitoring individual speakers is critical, speaker identification is necessary.
Labelling voice segments with emotions or sentiment categories is a component of sentiment analysis for audio data. Understanding the emotional content of audio recordings, such as recognising happiness, sorrow, rage, or neutrality, is much easier by completing this exercise. Sentiment evaluation is essential.When it comes to offering suitable replies or individualised services, applications like customer feedback analysis, social media monitoring, or voice-activated virtual assistants, sentiment analysis is essential.
In audio analysis, transcription and labelling of sound occurrences or background noises are also crucial. This entails labelling audio data with descriptions of certain sound occurrences or classifications, such dog barking, automobile horns, or bird chirps. Applications like soundscape analysis, acoustic monitoring, and ambient sound recognition all depend on the detection and labelling of sound events.
The proficiency of the annotators, the accessibility of high-quality annotated datasets, and the ongoing development of annotation methodology all contribute to the effectiveness of audio labelling. The performance of is directly impacted by the labels' uniformity and correctness.
The performance of downstream applications, such as building strong machine learning models or offering insightful analysis of the labelled audio data, is directly impacted by the precision and consistency of the labels.
In conclusion, audio labelling is a crucial step that enables the extraction of important knowledge and insights from audio data in a variety of fields. Accurate and consistent labelling is essential for creating trustworthy systems and applications, whether it be for voice transcription, speaker identification, emotion annotation, or labelling sound occurrences. Audio labelling allows improvements in voice recognition, speaker identification, sentiment analysis, transcription services, and sound event detection through meticulous data collection, clear annotation criteria, efficient annotation techniques, and quality control procedures. Audio labelling approaches will advance as technology develops, spurring innovation
2. Data Annotation Services
The need for data annotation services in India has been rising quickly as a result of developments in machine learning (ML) and artificial intelligence (AI) technology. To meet the demands of sectors including healthcare, banking, e-commerce, automotive, and more, a number of businesses in India provide data annotation services. By assisting businesses in producing high-quality labelled datasets, AI and ML models may be trained and improved.
India's data annotation service providers provide a wide range of annotation features, including the ability to annotate text, audio, video, images, and sensor data. These services require work that includes speech-to-text transcription, object identification, semantic segmentation, picture classification, and more. Teams of experienced individuals educated in certain annotation techniques often make up the annotation team
The presence of a sizable pool of talented and technically adept people is one benefit of outsourcing data annotation services to India. India has a robust IT sector and a large talent pool of data scientists, engineers, and annotation specialists who are skilled in properly annotating data.
Another benefit of using Indian data annotation services is their affordability. When compared to many other nations, India has a significantly cheaper cost of labour, which makes it a desirable alternative for companies wishing to outsource data annotation activities while upholding quality requirements.
To safeguard the privacy and safety of client data, data annotation service providers in India frequently have a strong infrastructure and secure data management procedures. These service providers place a high premium on adhering to data privacy laws including the General Data Protection Regulation (GDPR).
Large IT firms, specialised AI/ML startups, and research organisations are a few well-known data annotation service providers in India. To meet the specific needs of diverse sectors, these businesses provide scalable annotation solutions to manage both small- and large-scale annotation projects.
In conclusion, there has been a huge increase in demand for high-quality labelled datasets in AI and ML applications, which has led to a boom in the data annotation services industry in India. India boasts a competent labour force, an efficient economy, a strong infrastructure, and a dedication to data protection.
3. Some Services available in India
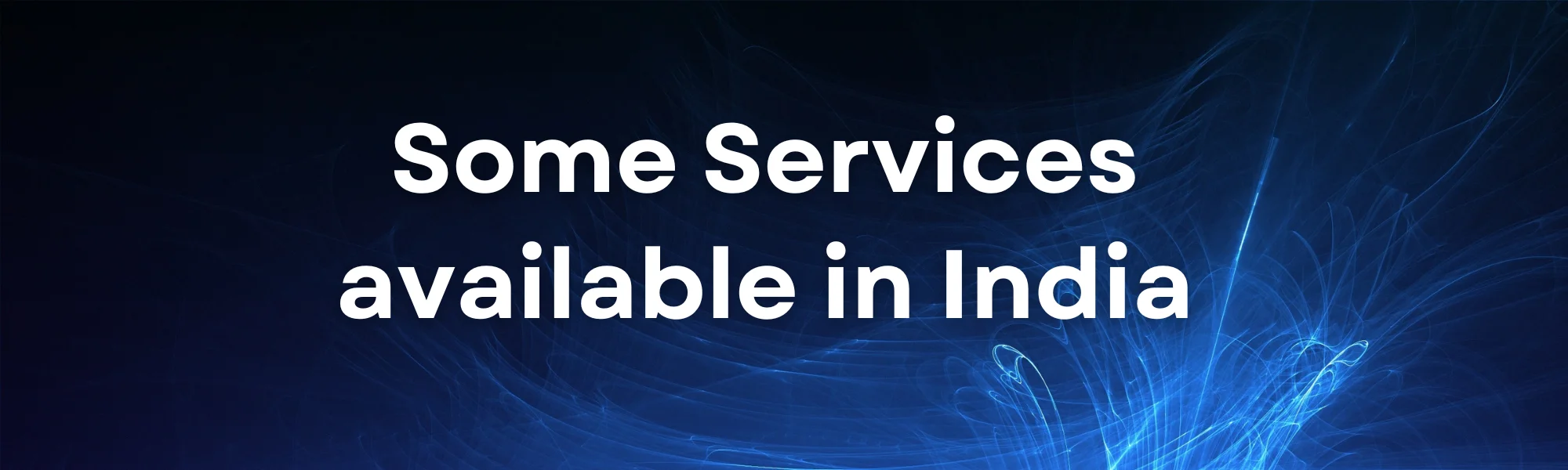
-
Cogito Tech LLC: Cogito offers a wide range of data annotation services, including image annotation, video annotation, text annotation, sentiment analysis, chatbot training, and more. They specialize in AI and ML-based solutions and cater to industries like healthcare, e-commerce, autonomous vehicles, and robotics.
-
Playment: Playment provides scalable and high-quality data annotation services, focusing on computer vision tasks such as image and video annotation, object detection, semantic segmentation, and 3D point cloud annotation. They serve industries such as autonomous driving, agriculture, retail, and augmented reality.
-
Appen: Appen is a global leader in data annotation services, including image, video, and audio annotation, sentiment analysis, speech recognition, and natural language processing. They have a strong presence in India and offer flexible and customizable annotation solutions for various industries.
-
iMerit: iMerit is a data annotation service provider known for its expertise in image and video annotation, natural language processing, and speech recognition. They offer comprehensive annotation solutions for industries like healthcare, e-commerce, fintech, and social media analysis.
-
Plutoshift: Plutoshift specializes in data annotation services for industrial applications, with a focus on IoT sensor data annotation. They provide annotation solutions for machine condition monitoring, predictive maintenance, and industrial process optimization.
-
Axtria: Axtria offers data annotation services for the healthcare and life sciences industry. Their expertise includes clinical data annotation, pharmacovigilance, adverse event detection, and drug safety monitoring.
-
DefinedCrowd: DefinedCrowd provides data annotation and data enrichment services for machine learning models. They offer image annotation, text annotation, speech annotation, and natural language processing annotation solutions for various industries.
-
These are just a few examples of data annotation service providers in India. It's important to conduct thorough research and evaluate their specific services, expertise, and industry focus to find the best fit for your data annotation requirements.
4. What is Data Annotaion in Machine Learning
In order to construct a training dataset or ground truth for machine learning models, data annotation—a critical step in the field—involves labelling or categorising data. It is a crucial stage in supervised learning, in which models learn from labelled examples to generate predictions or categorise unknown data. Data annotation gives machine learning algorithms the context and details they need to recognise and interpret patterns, connections, and characteristics in the data.
The process of annotating data often requires human specialists or annotators who meticulously examine and label each instance of data. Depending on the job and topic, several labels may be used, ranging from straightforward binary classifications (such as positive/negative) to more intricate multi-class categorizations or hierarchical annotations. For tasks like as object recognition or picture segmentation, the annotations might also contain bounding boxes, polygons, or segmentation masks.
Image, text, audio, video, and sensor data annotation are a few of the several forms of data annotation that are frequently utilised in machine learning. Each kind of annotation has its own distinct problems and solutions. We shall examine a few of the machine learning techniques for data annotation.
Labelling or highlighting particular areas or objects inside an image is known as image annotation. Object identification, where bounding boxes are formed around things of interest, or semantic segmentation, where pixel-level annotations are supplied to identify the borders of various objects in a picture, are examples of jobs that fall under this category. Keypoint annotation, which labels certain locations of interest within an image, is another type of image annotation.
Adding labels or tags to textual data, such as phrases, papers, or paragraphs, is known as text annotation. It entails activities like named entity recognition, in which the text is searched for and labelled with certain entities like persons, places, or organisations. Another text annotation task is sentiment analysis, in which text segments are given sentiment labels (such as positive, negative, or neutral)
Labelling or transcription of spoken words or noises inside an audio recording is known as audio annotation. This is frequently applied for trancribing audible words into written text, as in voice recognition tasks. Another audio annotation assignment is speaker identification, which involves labelling and identifying the various speakers in an audio recording. Audio data may also be annotated with emotions by categorising certain voice segments according to emotions or sentiments.
Labelling or annotating objects, activities, or events in a video sequence is known as "video annotation." This may entail activities like object tracking, in which items of interest are identified and tracked across several frames, or activity recognition, in which certain behaviours or activities are identified within the video. Another video is one that identifies faces.
Another video annotation task is facial recognition, which involves finding and labelling faces in order to identify people in the film.
Annotating sensor data entails labelling or tagging information gathered from a variety of sensors, including GPS, accelerometers, and gyroscopes. Applications like activity monitoring, where human behaviours are labelled based on sensor data, frequently employ this kind of annotation. In sectors like robotics and autonomous vehicles, where sensor inputs must be labelled for training models to make educated judgements, annotation of sensor data is also essential.
To guarantee accurate and consistent annotations, the data annotation process needs rigorous preparation, precise annotation criteria, and quality control techniques. The standards, regulations, and classifications that annotators adhere to while giving labels are laid forth in annotation guidelines.
To promote uniformity among annotators and ensure high-quality annotations, these rules must be clearly stated and plain.
Inter-annotator agreement is one of the quality control tools used to quantify the degree of agreement between various annotators. In this scenario, the same piece of data is independently annotated by a number of people, and any conflicts are settled by adjudication or debate. To maintain annotation quality and handle any problems or ambiguities that may develop throughout the annotation process, regular feedback, training, and calibration sessions are held.
Particularly for large-scale datasets, data annotation may be a time-consuming and labor-intensive operation. Automated annotation methods, such active learning and semi-supervised learning, have been developed to deal with these problems.
By identifying just the most insightful cases for annotation, these strategies seek to optimise the annotation process while requiring less work overall.
Data annotation duties are increasingly being outsourced to specialised service providers in recent years. These businesses have infrastructure and specialised personnel to handle enormous amounts of data annotation effectively. To offer accurate and scalable annotation services, they frequently use a combination of human and machine annotators.
For the creation and use of machine learning models to be effective, high-quality annotated datasets must be available. Models use annotated data as a training set to discover patterns and make precise assumptions about unobserved data. But it's important to understand that data annotation is a continuous process.
Iterative re-annotation or continuous annotation may be required to update and improve the training datasets as models get better and new data becomes available.
An important step in machine learning that enables models to learn from labelled instances is data annotation. It entails the labelling or annotation of data instances across several domains, including pictures, text, audio, video, and sensor data, by human annotators or specialists. To guarantee correct and consistent annotations, careful preparation, unambiguous instructions, and quality control procedures are essential.
The need for labelled datasets is growing, and to meet the problems of scalability and efficiency, the area of data annotation is continuing to develop with automated annotation techniques and the introduction of specialised service providers. The creation and use of reliable and effective machine learning models heavily depend on the accessibility of high-quality annotated datasets.
5. Data Annotaion Tools
The labelling or annotating of data for machine learning tasks is facilitated by the use of software platforms or programmes called "data annotation tools." To simplify the annotation workflow and increase the effectiveness and accuracy of the annotation process, these solutions offer a user-friendly interface and a variety of annotation features. In this section, we'll look at various popular data annotation tool types and their characteristics.
Object identification, semantic segmentation, and picture classification are just a few of the tasks that may be annotated images for using the tools for image annotation. These tools frequently have capabilities like the ability to draw bounding boxes, polygons, or masks around interesting items. In order to expedite the annotation process, some solutions additionally offer automated annotation features like pre-trained models for object recognition.
Text Annotation Tools: Text annotation tools are used to label or tag textual material in order to perform tasks like sentiment analysis, named entity identification, or text classification. These tools include features like text segment or entity highlighting, text labelling or category assignment, and the ability to design unique annotation schemas. Additionally, they could have tools for version control, inter-annotator agreement analysis, and collaborative annotation.
Tools for Audio Annotation: Tools for audio annotation may be used to mark up audio data for functions like emotion recognition, speech recognition, and speaker identification. With the use of these technologies, users may categorise certain audio segments or emotions as well as identify speakers and transcribe spoken phrases. For the purpose of making the annotation process easier, they frequently provide audio playback and visualisation options.
Tools for annotating videos include object tracking, activity detection, and facial recognition. These tools are specifically designed for annotating video data. These features enable users to identify actions or events, draw bounding boxes or polygons around objects, and monitor objects across numerous frames. Additionally, they could include tools for frame-level annotation and synchronisation with sensor or audio data.
Tools for annotating sensor data include accelerometers, gyroscopes, and GPS sensors. These tools are used to annotate sensor data that has been acquired from a variety of sources. With the use of these technologies, sensor data from applications like activity monitoring, robotics, or autonomous cars may be annotated. Specific actions, occurrences, or abnormalities within the sensor data streams can be labelled by users.
There are extensive annotation systems that handle a variety of data formats and annotation jobs in addition to task-specific annotation tools. These systems frequently provide quality control methods, collaborative annotation tools, and procedures that may be customised. They could have tools for organising annotation projects, delegating work to annotators, and keeping track of development. To help with the annotation process, some systems additionally include automated features like active learning or pre-trained models.
Labelbox, RectLabel, VGG Image Annotator (VIA), Prodigy, LabelImg, OpenLabeling, Anno-Mage, and many more are well-known systems and tools for data annotation. These tools come in a variety of features, usability levels, and price ranges. The exact annotation task, the type of data being annotated, the need for collaboration, and the budget all play a role in selecting the best data annotation tool.
It's important to note that certain technologies also interact with machine learning platforms or frameworks, making it possible to include annotated data into the pipeline for training models without any issues. This integration makes it easier to give annotated data to machine learning algorithms and makes it easier to train and upgrade models iteratively.
The data annotation process for machine learning tasks may be streamlined and improved with the help of data annotation tools. They provide user-friendly interfaces and a variety of annotation features that are suited to various data formats, including photos, text, audio, video, and sensor data. By increasing the process' speed, accuracy, and consistency, these technologies make it possible to produce labelled datasets of the highest calibre, which are then used to train and enhance machine learning models.
6. Conclusion
In conclusion, Medreck stands out as a provider of data annotation services due to their extensive experience, customized solutions, quality control measures, scalability, data security practices, collaborative approach, domain expertise, and timely delivery. Their commitment to delivering high-quality annotated datasets empowers clients to develop and deploy accurate and robust machine learning models. Medreck's expertise in data annotation makes them a reliable partner for organizations seeking to leverage annotated data for their AI and machine learning initiatives.